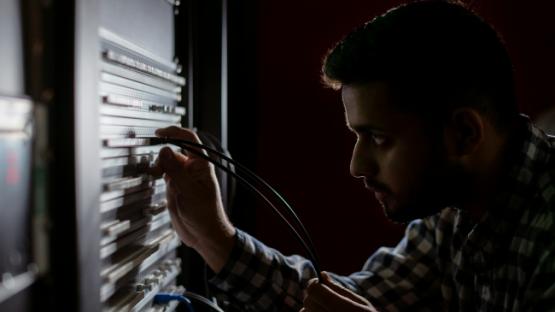
Photo by ThisisEngineering on Unsplash
This explainer takes a look at the main ways in which large language models (LLMs) threaten your privacy and data protection rights.
Photo by ThisisEngineering on Unsplash
The emergence of large language models (LLMs) in late 2022 has changed people’s understanding of, and interaction with, artificial intelligence (AI). New tools and products that use, or claim to use, AI can be found for almost every purpose – they can write you a novel, pretend to be your girlfriend, help you brush your teeth, take down criminals or predict the future. But LLMs and other similar forms of generative AI create risks – not just big theoretical existential ones – but ones which can harm people today.
In this explainer, we take a closer look at some of the risks for privacy and data protection that arise from the development and deployment of LLMs. LLMs (and other generative AI models) threaten privacy because they depend on the indiscriminate acquisition of large amounts of data and because they are unable to properly uphold the exercise of people's data subject rights.
LLMs are commonly trained to work as chatbots: they take inputs (‘prompts’) from users and provide structured and plausible responses that sound ‘human-like’ in their content and style. They do this by predicting what is the most likely word to follow a given body of text: their output is generated based on analysis of large amounts of text, the patterns within that text, and additional parameters set by developers or users.
Risks for privacy and data protection come from both the way that LLMs are trained and developed and the way they function for end users.
Developing a LLM requires enormous amounts of text to train the models: the more written language they can get hold of, the better. For companies developing LLMs, the primary source for this is of course the internet. Bots (specifically ‘web-scrapers’) can be programmed to download terabytes of online content from news sites, blogs, social media, anywhere they can reach. The data scraped can therefore include anything that is publicly accessible online, whether the company has the right to use it or not.
AI developers use both publicly available datasets of scraped data (such as Common Crawl or The Pile) and data they have scraped themselves. In some cases, companies have agreed a licensing deal to pay to be able to collect this data, such as between OpenAI and Reddit.
Inevitably we don’t know exactly what data most (but not all) LLMs are trained on. Open AI, Google, Meta and the like are generally pretty secretive about this. But it’s a pretty safe bet that this has included both personal data and copyrighted content (such as the Book3 subset of data in The Pile), and we know that companies are quietly changing their privacy policies to expand what data can be used for AI training. That’s a huge problem. Not just because sensitive or personal information might be scraped up and then regurgitated by the models (see below), but also because the people who wrote or provided that content didn’t know about or agree to it being used in that way. That flies in the face of data protection principles.
Data protection laws – like the GDPR – require companies to have a legal justification (a lawful basis) for collecting and processing personal data. The only potentially applicable lawful basis for training LLMs is to argue that doing so is a ‘legitimate interest’ of the company, that the processing is necessary to that legitimate interest, and that the interests and fundamental rights and freedoms of people whose personal data is processed are not overridden.
This requires a careful and thorough assessment – one that we don’t think indiscriminate scraping for LLM training can meet. Not least because LLM training is not widely understood and certainly would not have been expected before 2022, when much of the scraped data was produced and made available online. The fact that LLMs can be used for all manner of tasks – and cause all manner of harms – means that no one can do a genuine assessment of whether the rights and freedoms of individual people whose data is scraped, processed and regurgitated can be impacted.
Because training data is enmeshed in LLM algorithms, it is possible for this to be extracted (or ‘regurgitated’) by feeding in the right prompts. The New York Times have brought a legal challenge against Open AI because they have shown that the right prompts result in their copyrighted content being spat out. And DeepMind researchers found a way to get hold of potentially sensitive personal data through more absurd techniques.
Prompt engineering is an accepted part of LLM interaction – so it’s no surprise that many attempts are made to ‘jailbreak’ LLMs. These jailbreaks can also exploit the fact that user inputs can interact directly with the system producing the output to manipulate it. The result is that people are essentially able to make unpredictable data lookups to LLM databases – and control over what is outputted is minimal. The possibility to use LLMs (in particular ones that have been made available with open source weights) to make deepfakes, to imitate someone’s style and so on shows how uncontrolled its outputs can be.
Under data protection law, you have rights that you can assert over data related to you. In particular, you have:
However, LLMs are unable to adequately uphold these rights. Because information about you is held within the parameters of a model in addition to more traditional form (such as a database), it’s not obvious how AI companies can identify, correct or delete that information. And because input/output filters are always susceptible to ‘jailbreaking’ or ‘prompt injection’, they are unable to adequately prevent personal data from being outputted (which can be harmful whether the information is true or hallucinated).
While people can seek to block automated scrapers on their website or content, or request LLMs not to use data you input to train their models, such methods have limited efficacy, can be bypassed and were mostly unavailable when the first LLM-powered products launched. This makes it clear that many people’s rights may have been infringed in the training of existing generative AI models.
A fundamental principle of data protection law is ‘data minimisation’. This means that you shouldn’t be using more data than is necessary to achieve your goals. This flies directly in the face of LLM training, which to date has largely been an exercise in ‘data maximisation’. While AI tools can be made with smaller datasets, the norm is to go big.
The very thrust of any business model which is premised on the idea of collecting and processing as much data as possible is not only inherently risky for people’s privacy, but potentially runs afoul of data protection law. In prioritising quantity over quality, generative AI also jeopardises another fundamental principle of data protection law: accuracy.
LLMs are meant to be multi-purpose. That’s the whole point – they can be used to help with recipe ideas, writing software code, and to provide medical advice. This poses a problem for AI developers. It means that – by definition – they can’t explain exactly why they want to collect this data and what it will be used for. But a key tenet of data protection law is that a company needs to be clear about what the purpose is for its collection and processing of data. The generality (and vagueness) of LLMs has the effect of moving the goalposts and reducing certainty and clarity for people whose data is being processed.
Douwe Korff has criticised a “two-phase process” that allows data to first be used to train a general purpose LLM, and then be used subsequently for a more specific purpose (such as summarising legal documents).
Another source of training data for LLMs is the prompts inputted by users interacting with LLM-powered products like AI chatbots. It’s entirely possible that highly sensitive and private information has been fed into AI models since they were made available to the public in November 2022. Relying on user inputs (and feedback on the responses received) to finetune results is done by search engines and LLMs alike.
Again, people might not be aware that the questions they are feeding to LLM-based AI products can be absorbed into their datasets for further training of the underlying model. Governments have been quick to issue guidance instructing staff to be careful when using these tools when handling data about people or confidential information. And it was only after intervention by the Italian Data Protection Authority that OpenAI gave people the option to opt-out of their interactions being used to train. The problem is that once the data is in a model, it’s hard to get it out.
Privacy is also at risk with LLMs because of their ability to make inferences from the large amount of data available. Researchers at SRI Lab have made a tool that demonstrates how easily LLMs can work out personal information about you.
Because of the very large datasets involved, their combination may end up revealing much more about people than each underlying dataset alone.
LLMs’ operation is fundamentally based on analysis of large amounts of data. This inevitably creates risks and threats to principles of data protection and people’s rights. PI is watching closely how practice and regulation develop in this field. We're concerned that the majority of the large commercial generative AI models developed to date may have done so in violation of people’s rights under data protection law.